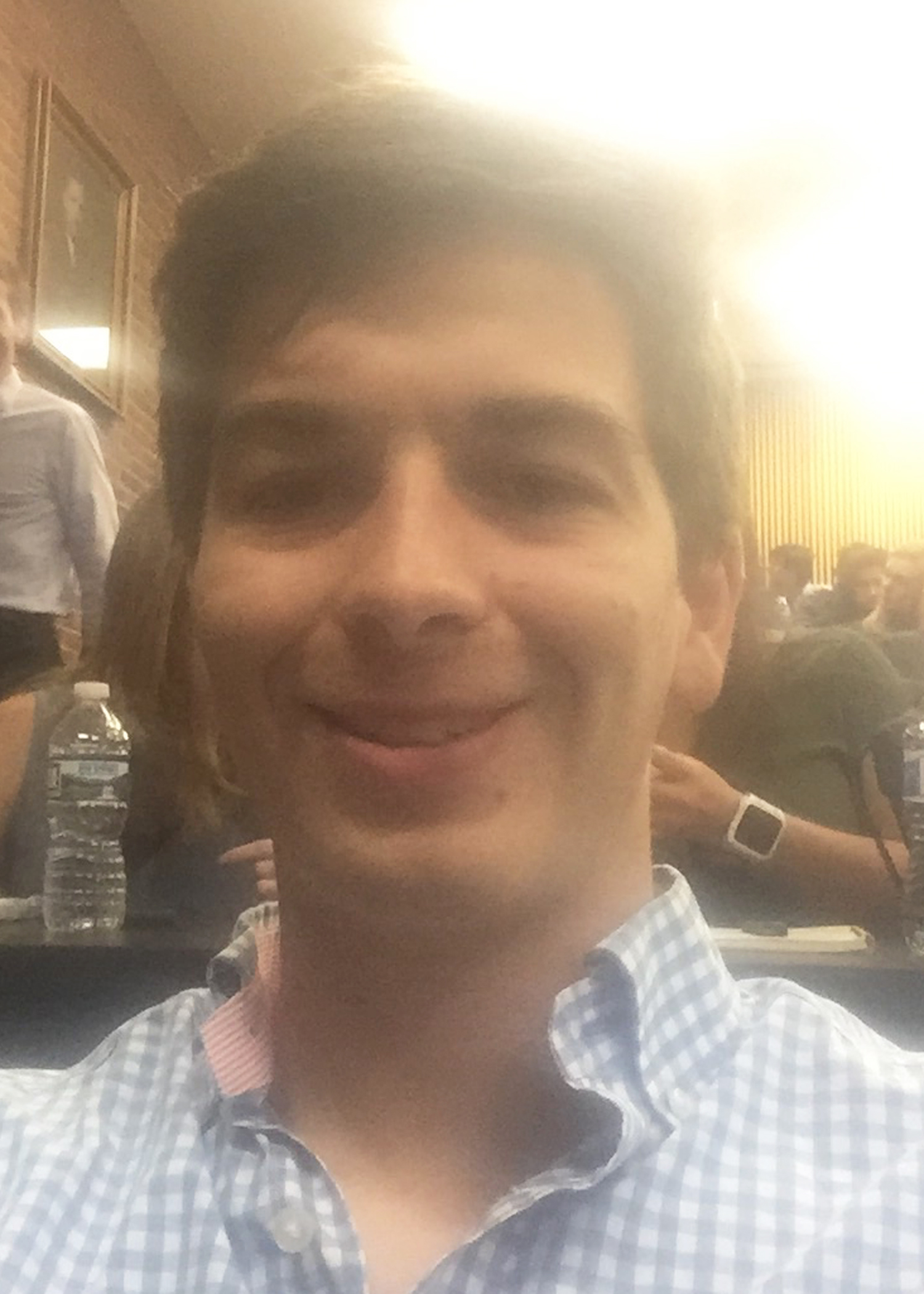
Clint Holt
PI: Ivelin Georgiev, PhD, Department of Pathology, Microbiology, and Immunology
Prediction of HIV Specificity from B-Cell Receptor Next-Generation Sequencing Data
Antibodies are crucial components in both antigen purification and validation, and can be ideal biological drugs due to high specificity and affinity for specific antigenic epitopes, high success rates for clinical trials, and long market lives. Although isolation and sequencing of B-cell receptor repertoires has become increasingly high-throughput in the last decade, the current methods of characterizing B-cell receptor specificities have remained relatively slow and expensive. The purpose of this work is to produce a prediction method for determining B-cell receptor specificity for the 6th most deadly disease worldwide, HIV. The set of all non-redundant 3-dimensional structures of antibody-HIV complexes from the Research Collaboratory for Structural Bioinformatics (RCSB) database (n=81) was used to generate weighted parameters for each residue in the B-cell receptor based on the frequency of interaction with HIV. These parameters will be incorporated into the creation of a machine learning classifier generated with a training set of several thousand HIV-specific and HIV-non-specific B-cell receptor sequences. Despite the majority of largely weighted residue parameters residing in the complementarity determining regions (CDRs), several key residues were identified in both heavy and light chains in the neighboring framework regions that will ideally enhance the classification accuracy of the machine learning model. With the increasing ease of generating biological data, machine learning models act as a great means to make predictions of a known confidence interval to influence selection of samples for subsequent research.