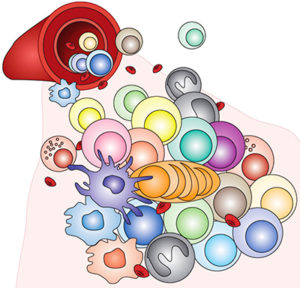
Cytomics, the “omics” of cell identity, offers the opportunity to systematically identify all cells in a tissue or patient sample, and the recent advent of high-dimensional flow and mass cytometry to the cytomics arsenal has markedly increased its power. Cytomics is particularly valuable in the detailed analysis of immune cell populations, which are frequently complex and dynamic. However, the full potential of this approach will be realized only if it can be applied to the assessment of serial samples over time. To do so requires new, carefully selected data analysis techniques that can mine the large, highly complex datasets resulting from serial measurements. Now, Vanderbilt Basic Sciences investigator Jonathan Irish, his graduate student Allison Greenplate, and their collaborators address this challenge. Their primary goal was to devise an analytical approach that could: 1) evaluate stability versus plasticity of the system, 2) detect changes in abundance of cell subpopulations, 3) identify signature features of cell populations, and 4) detect new or unexpected cell types. To achieve this goal, they used a combination of three statistical tools, t-distributed stochastic neighbor embedding (t-SNE), the Earth Mover’s Distance (EMD) algorithm, and Marker Enrichment Modeling (MEM). They hypothesized that this combination of tools would enable them to monitor changes in immune cell populations in patients undergoing cancer chemotherapy, and they tested this hypothesis using three publicly available cytomics datasets. The first dataset was obtained from melanoma patients undergoing anti-PD-1 therapy, which boosts the immune response to tumor cells by blocking the PD-1 (programmed cell death protein 1) receptor on T cells. The results of their analysis revealed only subtle changes in immune cells in the blood of seven out of eight patients. However, marked changes in the eighth patient’s peripheral blood immune cells foreshadowed the later development of myelodysplastic syndrome, a possible side effect of the therapy. The second dataset was obtained from patients with acute myeloid leukemia undergoing chemotherapy. In this case, marked changes were observed in the peripheral blood immune cells of three out of five of the patients, and these changes could readily be explained as a response to therapy. The remaining two patients exhibited only subtle changes, a finding consistent with a poor therapeutic response. The third dataset was obtained from melanoma patients undergoing targeted therapy to block two oncogenic protein kinases (BRAFV600and MEK). In this case, the researchers observed significant changes in five subpopulations of T cells that were present in the tumors. Of particular interest was a subpopulation of T cells that was not present in the blood or tonsils of healthy donors but was found in other forms of cancer, including glioblastomas and renal cell carcinomas. Thus, the research demonstrated the ability of the analytic approach to identify clinically significant unexpected outcomes of therapy, distinguish patients who responded to therapy from those who did not, and identify novel tumor-related cell types. These findings open direct new avenues of research, and since the methods are not limited to the study of immune cells, it promises to facilitate the investigation of a wide variety of both physiologic and pathologic tissue samples in the future. The work is published in the journal Cancer Immunology Research [A. R. Greenplate et al., (2018) Cancer Immunology Research, published November 9 DOI:10.1158/2326-6006.COR-17-0692].