By Leah Mann
The Aug. 30 School of Medicine Basic Sciences Apex Lecture featured visionary biophysicist and alumnus John Jumper, BS’07. The event was co-sponsored by the recently established Center for Applied Artificial Intelligence in Protein Dynamics, with Jumper serving as the center’s inaugural speaker.
Even before Jumper began his address, the enthusiasm for his talk and appreciation of his work was palpable. Hassane Mchaourab, Louise B. McGavock Chair of the Department of Molecular Physiology and Biophysics, introduced Jumper to a packed house of students, faculty, and staff as “one of the pioneers of the brave new AI world.”
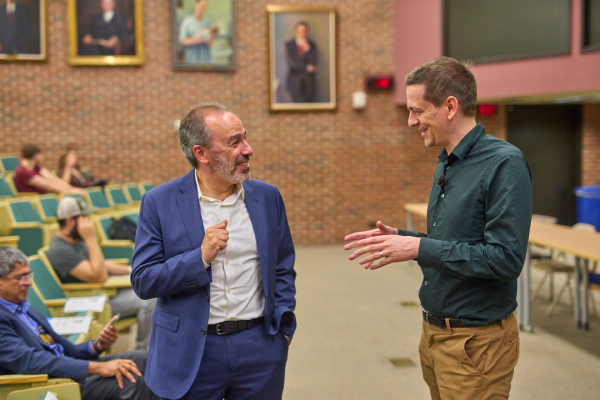
Jumper launched his journey to “[revolutionize] protein science” right here at Vanderbilt University, where he earned his bachelor of science in physics and mathematics. He received his master of philosophy in theoretical condensed matter physics from the University of Cambridge in 2008 and his doctor of philosophy in theoretical chemistry from the University of Chicago in 2017. Jumper then joined the artificial intelligence company DeepMind Technologies, where he now serves as a senior staff research scientist.
Jumper began his address by talking about AlphaFold, the revolutionary protein structure-predicting program. Jumper led its development at DeepMind, a subsidiary of Alphabet. Dubbing AlphaFold a “system for computational alchemy,” Jumper explained how the AI system tackled the age-old question of protein folding, or how amino acid sequences fold to form three-dimensional protein structures. The Protein Data Bank, which serves as the international repository for structural data, contains roughly 200,000 three-dimensional protein structures, but a single structure can take years to resolve using X-ray crystallography or cryogenic electron microscopy.
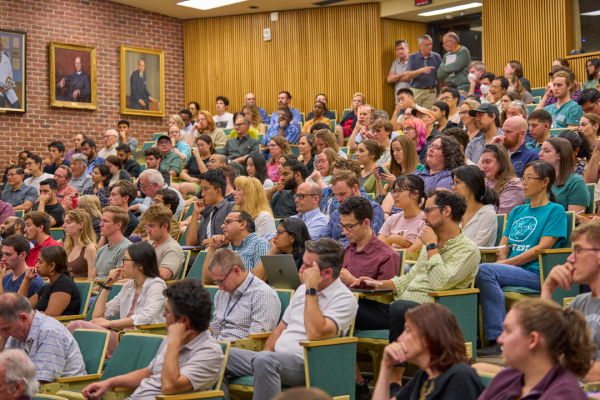
AlphaFold confronted this timely and expensive hurdle to determining protein structure. According to Jumper’s metaphor, AlphaFold thrives as an alchemist does when it has a “base ‘metal’ of protein sequence and you want to transmute it into the ‘gold’ of protein structure.” It functions as a deep learning tool, which means that it leverages layers of data to “learn” and boost performance.
Jumper and his colleagues trained AlphaFold on proteins that appear in the PDB, allowing them to make small changes to increase accuracy since both the input and output were known. However, their goal was to create a program that delivered more than just structure. They also needed to determine how well AlphaFold could predict a given region. While they were able to obtain confidence metrics as a coarse estimate of accuracy, Jumper noted that it’s not truly correct to say a structure is right or wrong, but that the confidence metrics should induce “some hypotheses you should believe, some hypotheses you should doubt.” Accordingly, the AlphaFold team decided to evaluate if “the position and orientation of one residue” was correct relative to another.
The final version of AlphaFold is the product of many changes, each improvement “[mattering] a bit and nothing [mattering] a lot.” Instead, the most significant development was the “methodology of building the protein intuition into the model.”
AlphaFold’s impact extends beyond that of structural biology, paving a path for chemists, pharmacologists, and biotechnicians to better understand protein function in the body and to improve drug discovery efforts.
Even more so, the success of AlphaFold spurs questions of working with AI to solve additional scientific problems. “We need to get better as a community at measuring how good our tools really are, where they fail, and when one tool is ever so slightly better than another,” Jumper said. “Then hopefully we can take sparse data in other fields and make it big data.”