The Idea
AlphaFold, the revolutionary artificial intelligence program developed by DeepMind (a subsidiary of Alphabet, Google’s parent company), uses machine learning to predict the 3D structures of proteins. Traditionally, protein structures are determined experimentally using methods such as x-ray crystallography or cryo-electron microscopy; however, proteins often adopt multiple conformational states, which may require multiple experimental structures over time to identify them. The development of new computational tools such as AlphaFold can cut down on the time needed to fully understand the relationship between a protein’s structure and function.
The AlphaFold software—now in its third iteration, AlphaFold3—has dramatically changed the field of structural biology. Yet not every tool is without its drawbacks, and new tools must be pushed and prodded to determine the full extent of their potential.
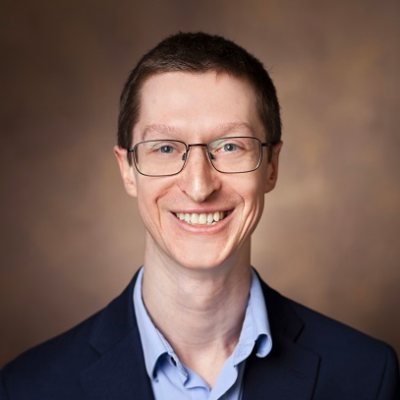
To this point, a group of School of Medicine Basic Sciences researchers, led by Benjamin P. Brown, assistant professor of pharmacology and member of the Center for Applied Artificial Intelligence in Protein Dynamics, set out to investigate the extent to which AlphaFold2 has learned biologically meaningful energetics of protein conformations.
The collaboration brought Brown together with Research Associate Professor of Molecular Physiology and Biophysics Richard Stein, Research Professor of Chemistry and Associate Professor of Pharmacology Jens Meiler, and Professor of Molecular Physiology and Biophysics Hassane Mchaourab and resulted in a publication in the Journal of Chemical Theory and Computation. Mchaourab, one of the corresponding authors, is the founding director of Vanderbilt’s Center for Applied AI in Protein Dynamics.
Why it matters
The likelihood that a protein will find itself in a given conformational state is directly related to the relative energetics of all accessible conformations. According to Brown, predicting which conformation a given protein will find itself in is extremely computationally expensive and scales poorly with the size of the system. The researchers strive to develop approaches that make inferring dynamic properties of proteins more computationally tractable.
To that end, Brown and colleagues used AlphaFold2 to see whether it had learned thermodynamic information, specifically as it relates to conformational free energies, and whether it could approximate the conformational flexibility of proteins.
The experiments yielded two main conclusions. First, even though the final output of AlphaFold2 is a static, non-moving structure, AlphaFold2 learns information that can suggest important dynamical properties of proteins. Second, AlphaFold2 is not entirely “mutation sensitive,” in other words, it does not necessarily correctly predict changes in the structure of a protein when a single amino acid is changed to a different amino acid. However, the researchers found that this does not mean that AlphaFold2 views each of those sequences identically, and that, in fact, it appears be sensitive to where in the sequence the mutation occurs and can provide functional insight such as which parts of a protein control its motion.
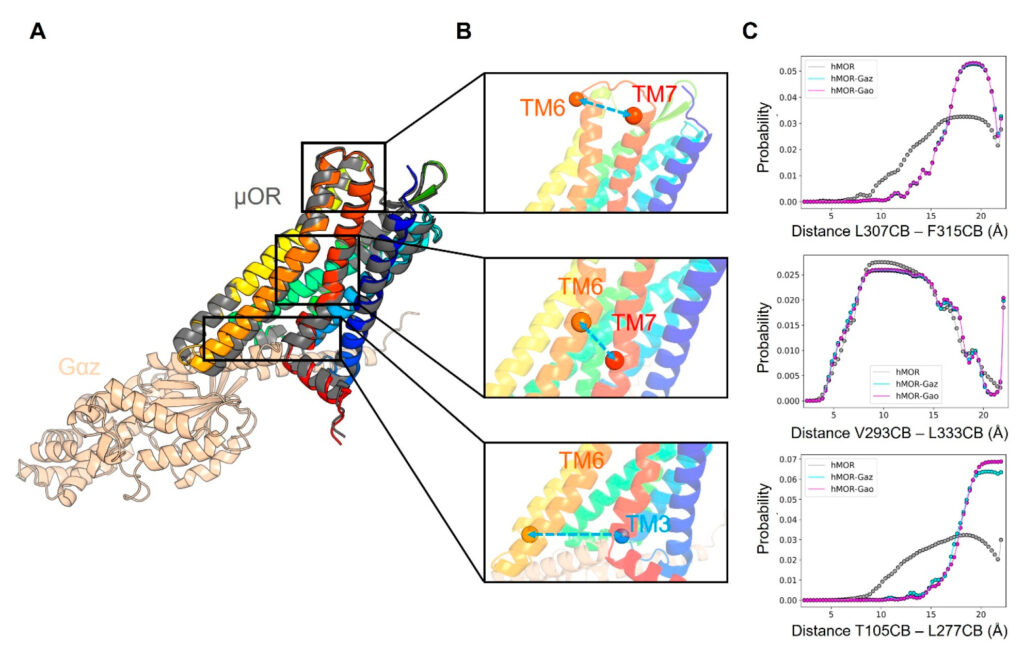
What’s next
A critical aspect of Brown et al.’s approach is that their results do not require the complete prediction of an entire protein structure using AlphaFold2, which can be deployed with less computational expense than mainstream methods such as molecular dynamics simulations.
“With this work, we hope that other researchers will use our approach to identify regions of proteins that are important for conformational dynamics, that impart allosteric function, and that may be susceptible to mutation-induced diseases,” Brown said. “We also hope that our research can help computational researchers identify collective variables for simulation and analysis.”
This manuscript is a substantial contribution toward the development of rapid methods to evaluate the functional properties of proteins. The refinement of artificial intelligence tools and the development of new applications of such tools that can be harnessed by increasing portions of the scientific community will open new research avenues that would otherwise have been prohibitively expensive or physically impossible for the average laboratory.
“AlphaFold3 was just released by Google DeepMind yesterday [May 9], and already people are sharing their insights into its potential,” Brown said. “Congratulations to the Google DeepMind team!. We look forward to seeing what impactful applications people derive from this next instantiation of AI in biomolecular modeling.”
Funding
Funding for this research was provided by the National Institute on Drug Abuse, the National Library of Medicine, the National Cancer Institute, and the National Institute of General Medical Sciences. Additional support was provided by the Alexander von Humboldt Foundation, the Deutsche Forschungsgemeinschaft, and the Federal Ministry of Education and Research in Germany. High-performance computing at Vanderbilt’s ACCRE facility is supported through the National Institutes of Health.
Go deeper
The paper “Approximating Projections of Conformational Boltzmann Distributions with AlphaFold2 Predictions: Opportunities and Limitations” was published in the Journal of Chemical Theory and Computation in January 2024.